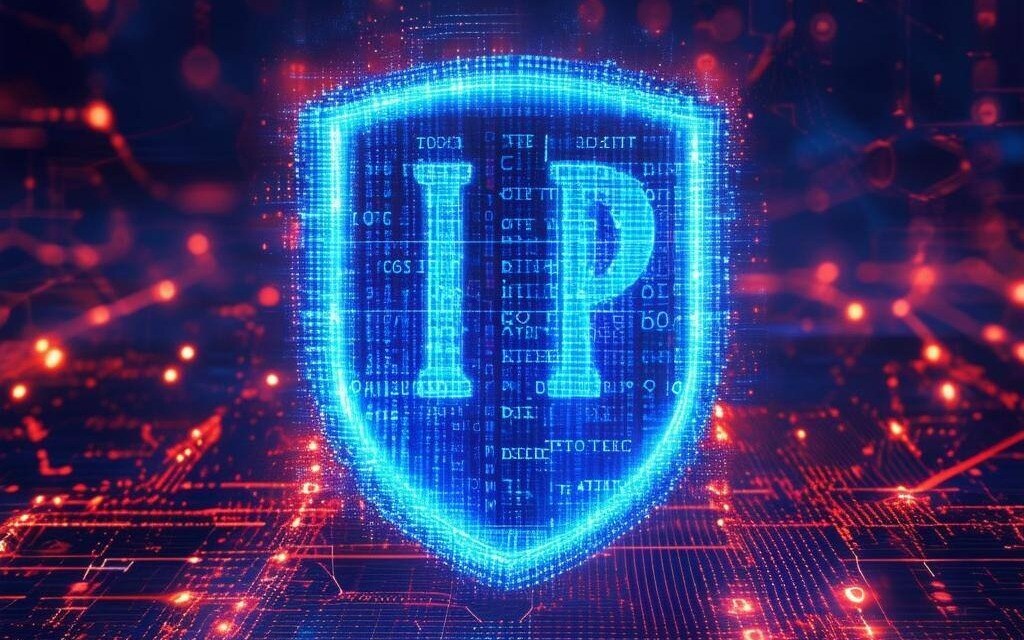
AI and Insider Threat: A Comprehensive Guide
Introduction:
In the rapidly developing landscape of digital technologies, Artificial Intelligence (AI) emerges as both a marvel and a challenge for organisations across various sectors. It automates mundane tasks and drives data-driven decisions, revolutionising how businesses operate. However, as we use AI to streamline operations and enhance security measures, we must also examine its role in facilitating and mitigating insider threats.
The need to understand this complex interplay is not just theoretical but urgent. With the increase of sophisticated technologies like Large Language Models (LLMs), organisations face new insider threat dimensions that need to be more understood and potentially require more control. This blog aims to introduce the dual role of AI in the sphere of insider threats, setting the stage for a deeper discussion on best practices and mitigation strategies.
The Shield: AI as a Preventative Mechanism
AI’s advanced algorithms can filter through vast amounts of data to identify patterns or behaviours within a network, flagging potential risks before they escalate into serious issues. For example, AI can be trained to recognise the signs of potential data exfiltration or anomalous login activities, thus serving as a proactive measure against internal threats.
One of the most effective tools in this arsenal is User and Entity Behaviour Analytics (UEBA). Sometimes also known as User Behaviour Analytics (UBA), these monitoring solutions utilise AI and machine learning algorithms to identify and halt behaviour that could signal an ongoing insider attack. For instance, a UEBA tool could instantly detect and disconnect a user who typically downloads only a small amount of data but suddenly starts downloading multiple gigabytes.
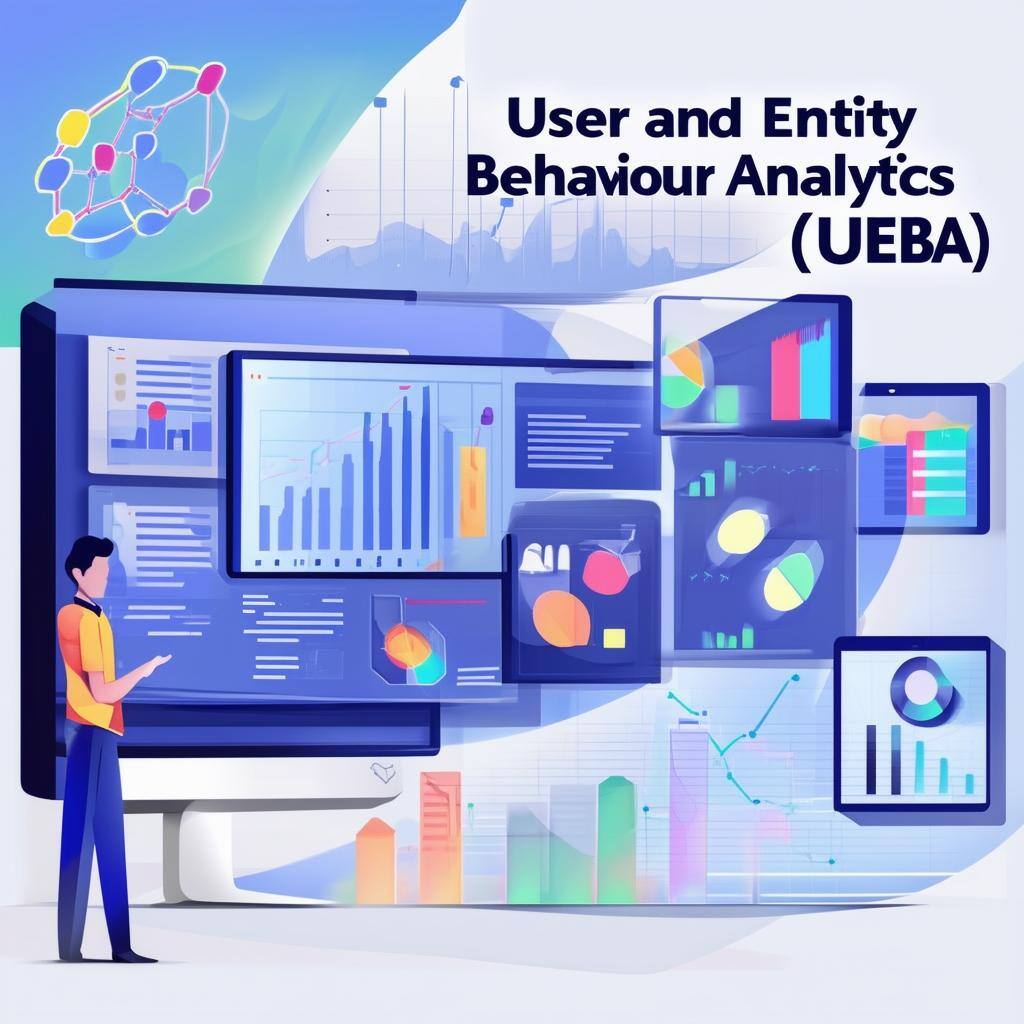
The Sword: AI’s Unintended Consequences
On the flip side, AI technologies, particularly LLMs like ChatGPT, have the potential to unwillingly facilitate insider threats. These models are becoming so advanced that they can generate human-like text. This text can then can be manipulated for malicious purposes such as social engineering or data theft. While LLMs are built to make tasks more efficient, in the wrong hands or without proper governance, they can serve as powerful tools for malicious or negligent intentions.
Additionally, employees might not be trained to handle AI adequately, especially when it comes to confidential information. Employees might unintentionally leak sensitive information to LLM’s which in turn can then present a threat to organisations. LLMs could potentially be hacked and the data be breached, therefore exposing organisational secrets or confidential information.
Five AI-Based Attacks Explored
In the following video, another dimension of AI’s potential risks will be explored. The video highlights five ways in which AI, specifically ChatGPT, can become a threat to your organisation’s security
Creating Governance for LLMs:
The rise of Large Language Models (LLMs) presents organisations with both opportunities and challenges. While outright bans on generative AI use within an organisation are generally unproductive, governance cannot be overlooked. Instituting robust governance protocols for LLMs involves several key steps:
- Data Sharing Restrictions: Define what types of data can and cannot be shared with AI. This can prevent sensitive information from being exploited.
- User Access Control: Establish who within your organisation has the authority to interact with LLMs and under what circumstances.
- Audit Trails: Maintain a detailed log of AI interactions to ensure traceability. This can be instrumental in investigating any potential insider threats.
By focusing on governance rather than prohibition, organisations can harness the advantages of AI while mitigating the risks.
Managing Insider Threats with AI: Strategies and Best Practices:
Effective insider threat management isn’t just about identifying potential risks; it’s about implementing strategies to mitigate them. Here are some best practices:
- Visibility: Ensure visibility over all data storages to prevent abuse or neglect of shadow databases.
- Data Classification: Classify all data based on its sensitivity and value to the organisation. Prioritise resources to secure the most critical data.
- Monitoring and Analytics: Utilise AI-driven analytics tools to monitor user behaviour and detect anomalies in real time. This could range from sudden privilege escalation to suspicious data movement.
The goal is to focus on data-centric security to answer key questions like who is accessing what, how, and from where. These best practices serve as building blocks for an effective insider threat management strategy, augmented by AI.
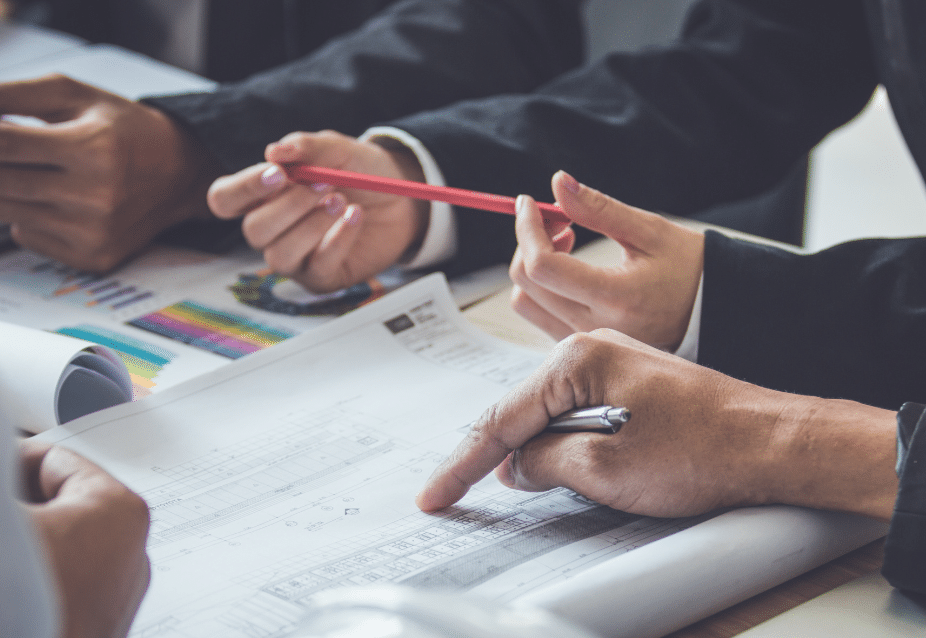
Real-life Implications and Case Studies
The Shadow of AI in Insider Risk
Research warns that the rising utilisation of large language models such as ChatGPT is almost certain to result in insider data breaches. Whilst organisations are tightening controls on what data can be shared with these chatbots, the same scrutiny is often absent when it comes to their own employees’ use of AI. Terry Ray, Imperva’s Senior Vice-President, highlights that an “overwhelming majority” of companies lack a strategy for insider risks. This lack of oversight leaves them vulnerable to unintended data leaks. These data leaks are caused by staff using AI to assist with tasks like coding or form-filling. Rather than prohibiting the use of AI, Ray argues that the focus should be on data access management. This includes cataloguing and classifying data, as well as employing data monitoring tools to spot any anomalous behaviour.
Costs of AI-Driven Efficiency
Another real-world example is the case of Summit Shah, the CEO of a Bangalore-based startup Dukaan. Summit Shah replaced 90% of his support staff with an AI chatbot, stating that it was both “tough” but “necessary.” While this move led to cost savings and efficiency gains for the company, it also potentially created an environment ripe for insider threats. Decisions like these can lead to a multitude of disgruntled employees, who may resort to malicious or negligent acts. In this case, while AI contributed to organisational efficiency, it also added a layer of complexity to the insider threat landscape. Read here why hostile work environments lead to increased insider threats.
Microsoft's Unintentional Insider
Similarly, an incident at Microsoft underscores how employees can unwittingly become insiders. A poorly configured URL within a GitHub repository dedicated to AI models unintentionally exposed 38TB of data. The data included sensitive information such as passwords and internal messages. Security provider Wiz highlighted that anyone with access to this URL could potentially inject malicious code into Microsoft’s AI models. Although the company swiftly rectified the leak, the incident serves as a cautionary tale. It shows that employees, in their quest for efficiency or innovation, can unintentionally pose a substantial risk to organisational data security.
Both examples underline the need for robust insider risk strategies that take into account both inadvertent and intentional misuse of technology. These incidents amplify the significance of a proactive approach to insider risks, particularly in this era of AI. Whether it’s the unregulated use of AI tools or an oversight in data access permissions, the risks are real and growing. Organisations should aim for a multi-layered security strategy centred on data access and behavioural analytics, keeping them one step ahead of potential threats.